In this activity, we will try to create a new Machine Learning model that will be able to identify and detect different types of hand poses and that can help us to control the Mecanum Pick and Place Robot. This activity can be quite fun and by knowing the process, you can develop your own customized hand pose classifier model easily!
We will use the same model that we have created in the previous Hand Pose Controlled Mecanum model to avoid any misdirection and confusion.
Note: You can always create your own model and use it to perform any type of functions as per your choice. This example proves the same point and helps you understand well the concept of Machine Learning models and environment.
Hand Gesture Classifier Workflow
Follow the steps below:
- Open PictoBlox and create a new file.
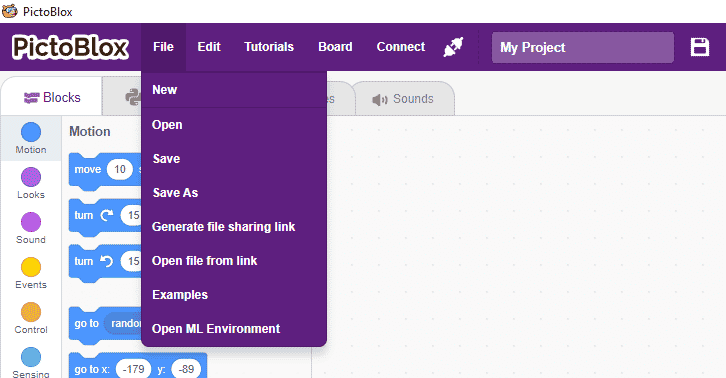
- Select the Block coding environment as appropriate Coding Environment.
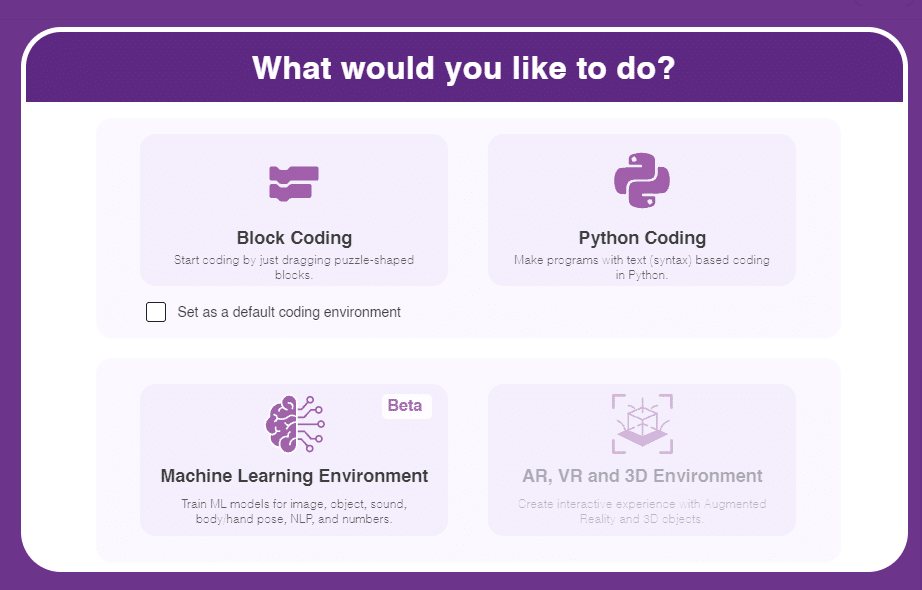
- Select the “Open ML Environment” option under the “Files” tab to access the ML Environment.
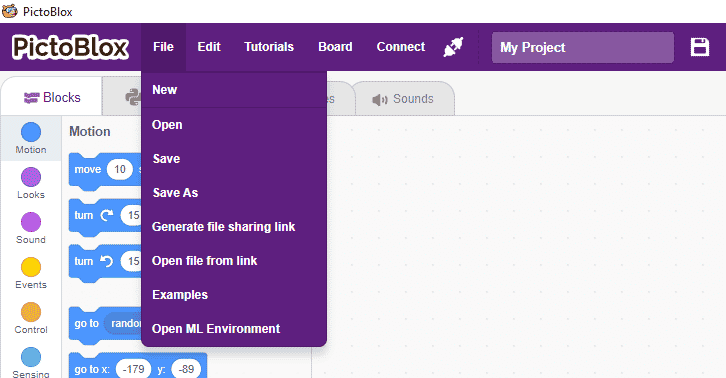
- Click on “Create New Project“.
- A window will open. Type in a project name of your choice and select the “Hand Gesture Classifier” extension. Click the “Create Project” button to open the Hand Pose Classifier window.
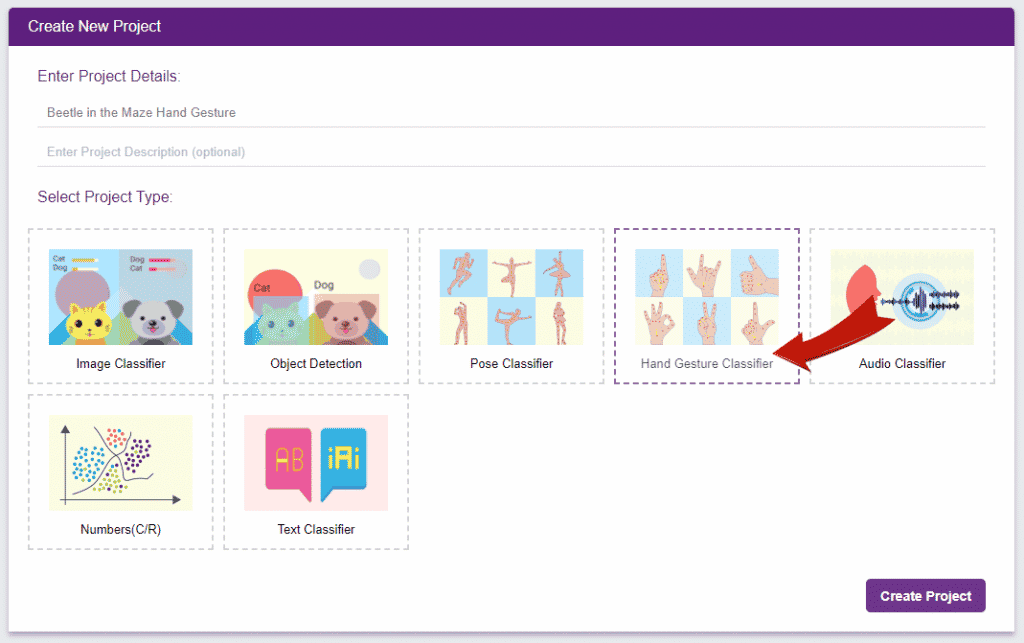
- You shall see the Classifier workflow with two classes already made for you. Your environment is all set. Now it’s time to upload the data.
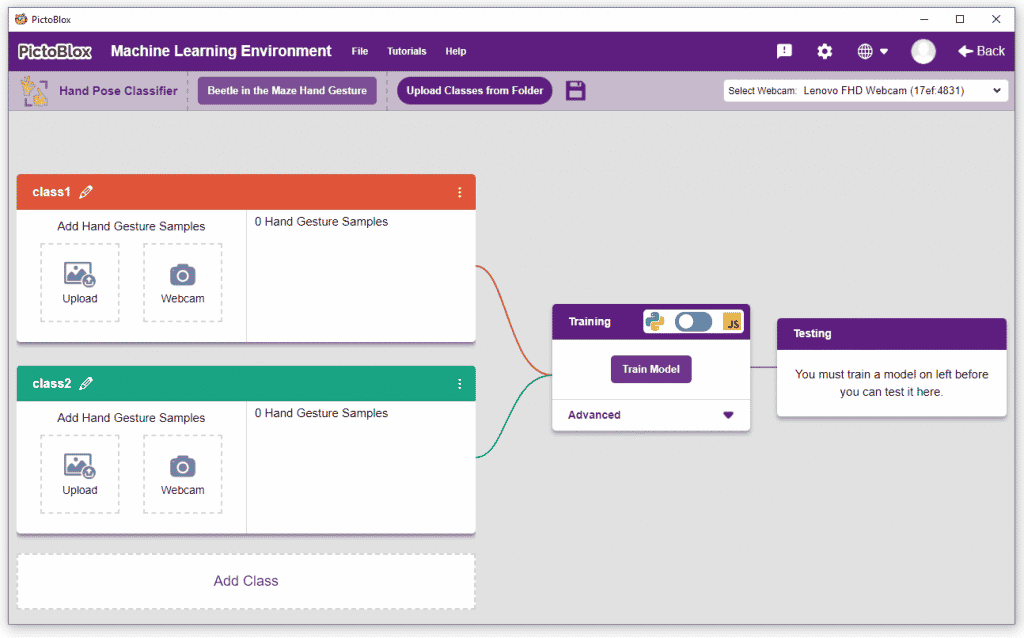
Class in Hand Gesture Classifier
There are 2 things that you have to provide in a class:
- Class Name: It’s the name to which the class will be referred as.
- Hand Pose Data: This data can either be taken from the webcam or by uploading from local storage.

Note: You can add more classes to the projects using the Add Class button.
Adding Data to Class
You can perform the following operations to manipulate the data into a class.
- Naming the Class: You can rename the class by clicking on the edit button.
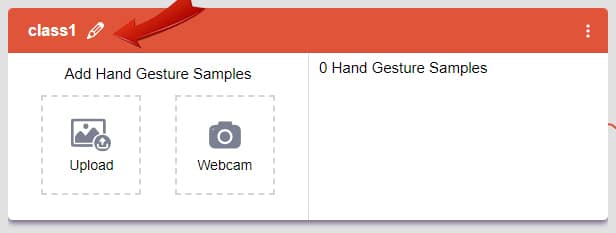
- Adding Data to the Class: You can add the data using the Webcam or by Uploading the files from the local folder.
- Webcam:
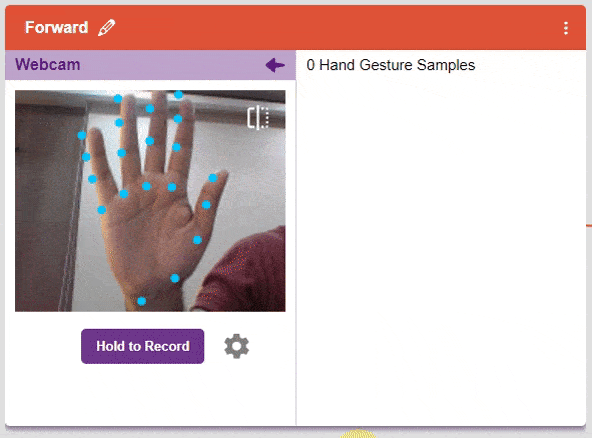
Note: You must add at least 20 samples to each of your classes for your model to train. More samples will lead to better results.
Training the Model
After data is added, it’s fit to be used in model training. In order to do this, we have to train the model. By training the model, we extract meaningful information from the hand pose, and that in turn updates the weights. Once these weights are saved, we can use our model to make predictions on data previously unseen.
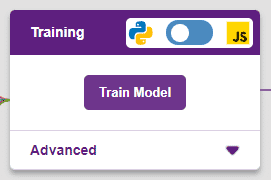
The accuracy of the model should increase over time. The x-axis of the graph shows the epochs, and the y-axis represents the accuracy at the corresponding epoch. Remember, the higher the reading in the accuracy graph, the better the model. The range of the accuracy is 0 to 1.
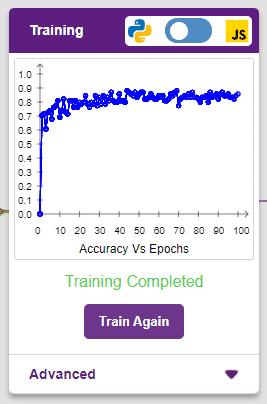
Testing the Model
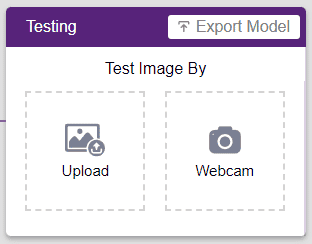
To test the model, simply enter the input values in the “Testing” panel and click on the “Predict” button.
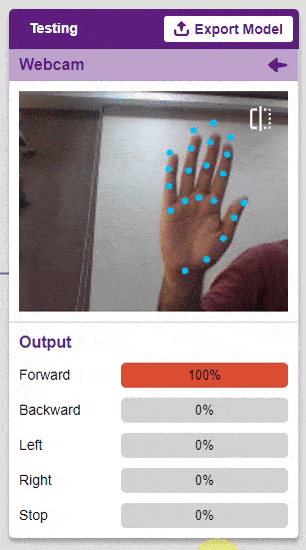
The model will return the probability of the input belonging to the classes.
Export in Block Coding
Click on the “Export Model” button on the top right of the Testing box, and PictoBlox will load your model into the Block Coding Environment if you have opened the ML Environment in the Block Coding.
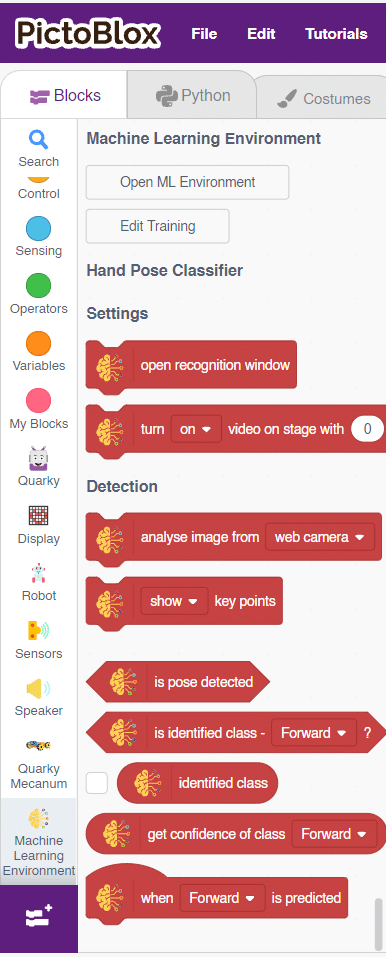
Logic
The mecanum will move according to the following logic:
- If the detected class is “forward”, we will make the Mecanum move forward.
- When the backward gesture is detected – Mecanum will move backwards.
- When the Lateral Left gesture is detected – Mecanum will move towards the left direction laterally with the help of its omnidirectional wheels.
- When the Lateral Right gesture is detected – Mecanum will move towards the right direction laterally with the help of its omnidirectional wheels.
- When the Normal Right gesture is detected – Mecanum will rotate on a single point towards the right direction.
- When the Normal Left gesture is detected – Mecanum will rotate on a single point towards the left direction.
- When the Stop gesture is detected – Mecanum will stop and initiate the Pick Mechanism using the Pick function.
- When the Circular Motion gesture is detected – Mecanum will initiate the Place Mechanism using the Place function.
Code
Initialization
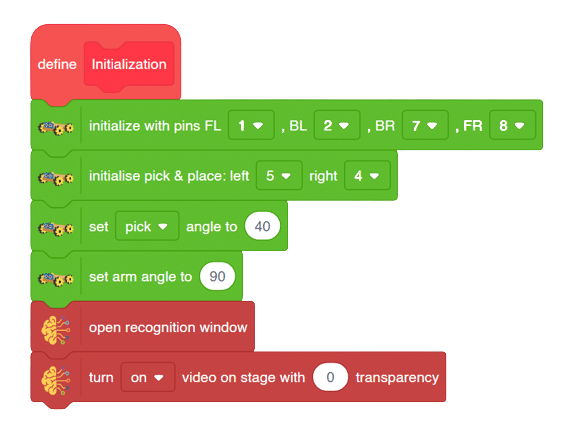
Main Code
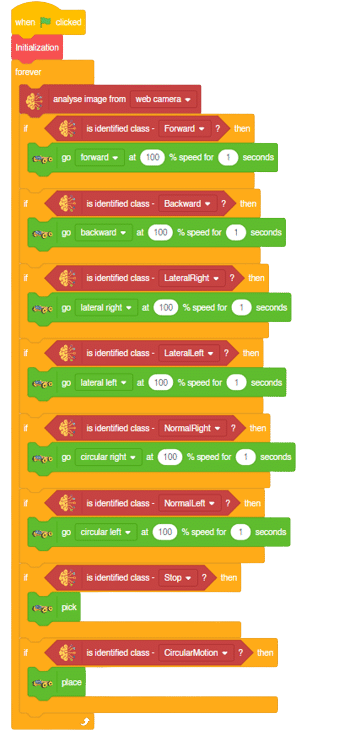
Output
Forward-Backward Motion:
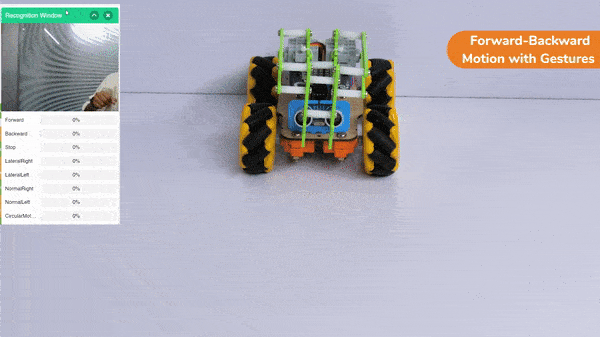
Circular Right-Left Motion:
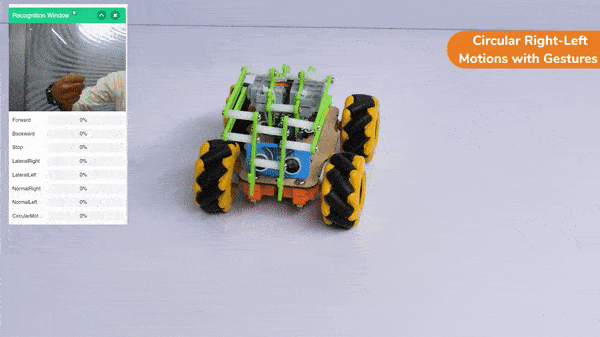
Lateral Right-Left Motion:
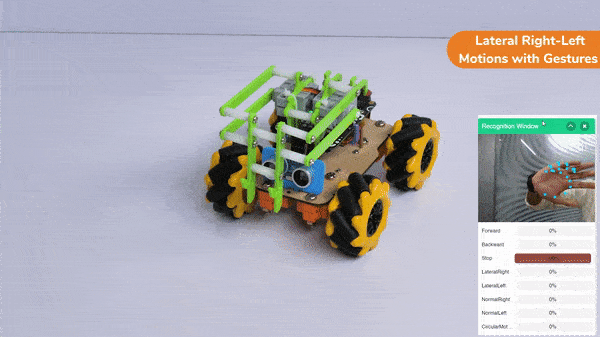
Pick and Place Mechanism with Hand Pose:
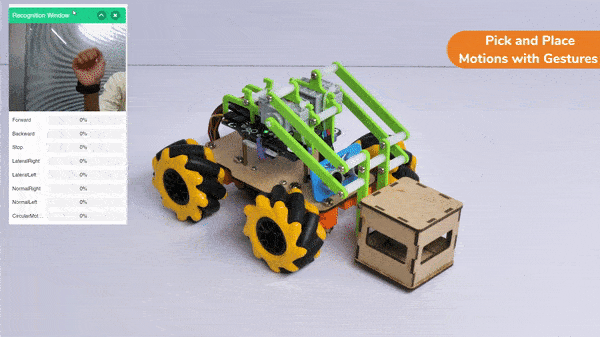