Introduction
This project demonstrates how to use Machine Learning Environment to make a machine–learning model that identifies the hand gestures and makes the Quadruped move accordingly. learning model that identifies the hand gestures and makes the qudruped move accordingly.
We are going to use the Hand Classifier of the Machine Learning Environment. The model works by analyzing your hand position with the help of 21 data points.
Hand Gesture Classifier Workflow
Follow the steps below:
- Open PictoBlox and create a new file.
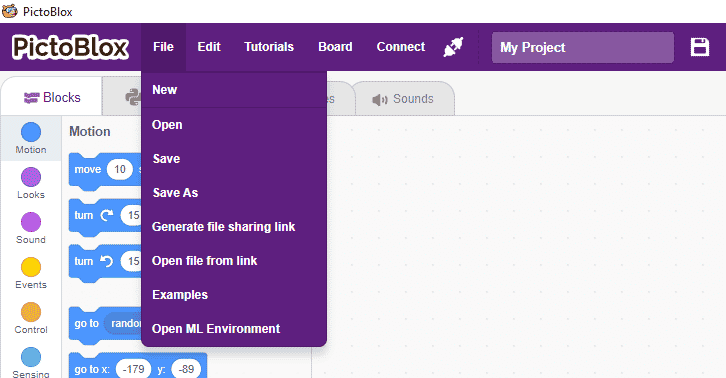
- You can click on “Machine Learning Environment” to open it.
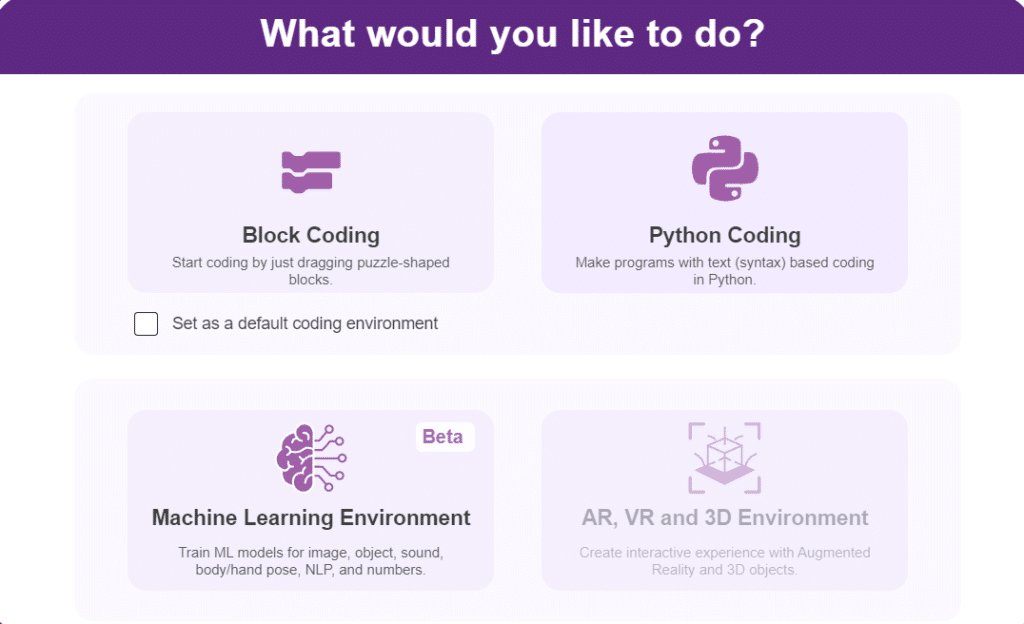
- Click on “Create New Project“.
- A window will open. Type in a project name of your choice and select the “Hand Gesture Classifier” extension. Click the “Create Project” button to open the Hand Pose Classifier window.
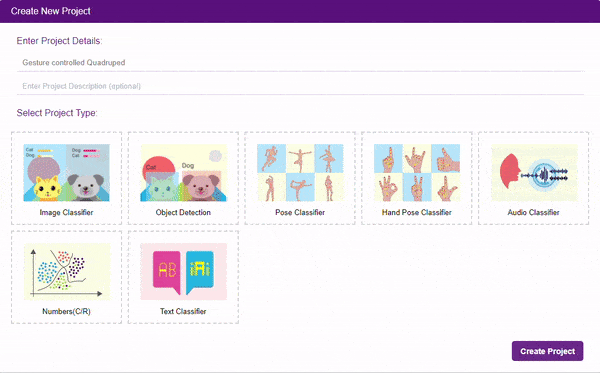
- You shall see the Classifier workflow with two classes already made for you. Your environment is all set. Now it’s time to upload the data.
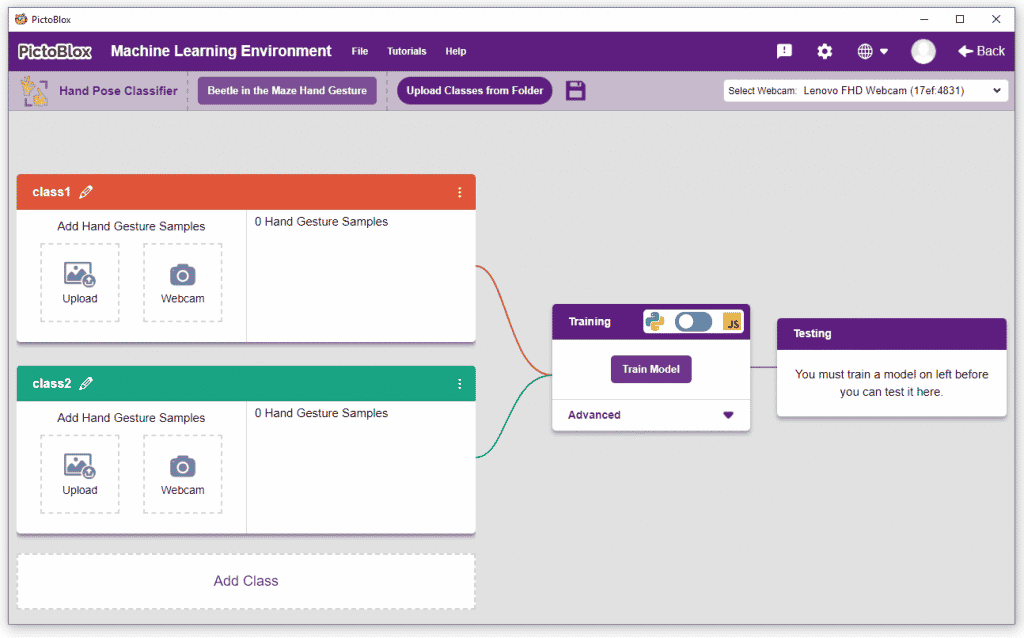
Class in Hand Gesture Classifier
There are 2 things that you have to provide in a class:
- Class Name: It’s the name to which the class will be referred as.
- Hand Pose Data: This data can either be taken from the webcam or by uploading from local storage.

Note: You can add more classes to the projects using the Add Class button.
Adding Data to Class
You can perform the following operations to manipulate the data into a class.
- Naming the Class: You can rename the class by clicking on the edit button.
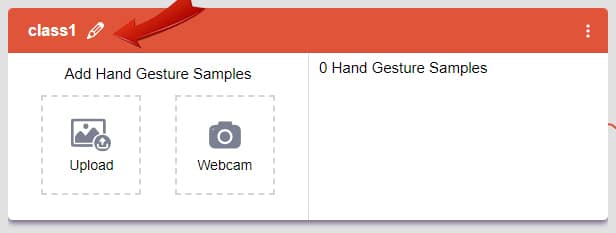
- Adding Data to the Class: You can add the data using the Webcam or by Uploading the files from the local folder.
- Webcam:
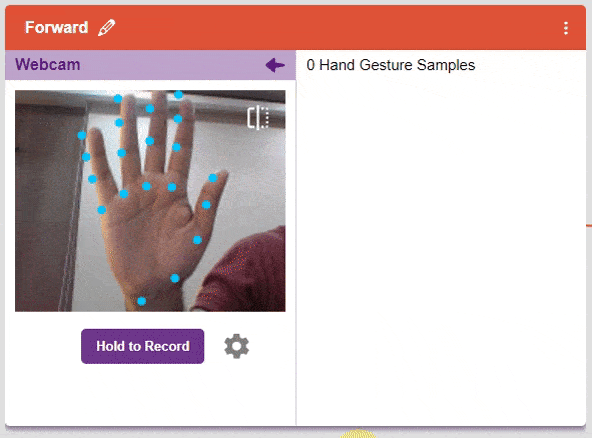
Note: You must add at least 20 samples to each of your classes for your model to train. More samples will lead to better results.
We are going to use the Hand Classifier of the Machine Learning Environment.
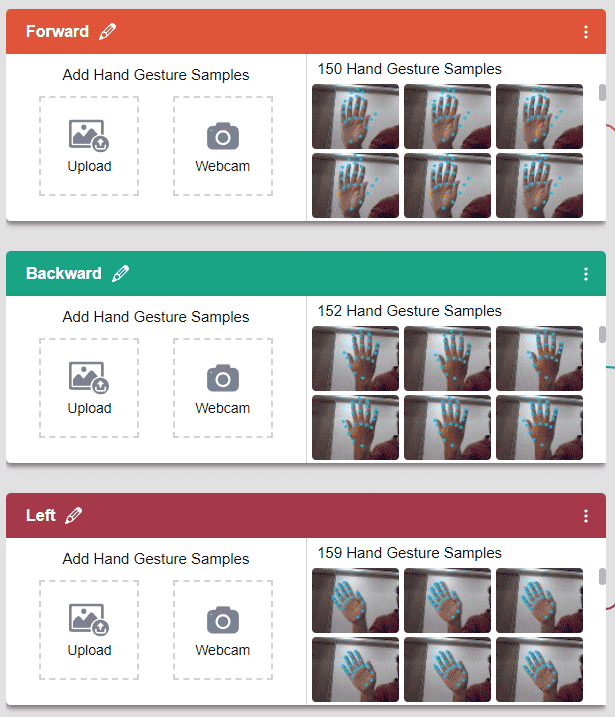
Training the Model
After data is added, it’s fit to be used in model training. In order to do this, we have to train the model. By training the model, we extract meaningful information from the hand pose, and that in turn updates the weights. Once these weights are saved, we can use our model to make predictions on data previously unseen.
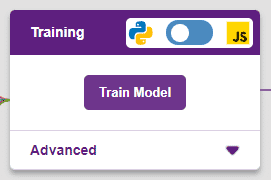
The accuracy of the model should increase over time. The x-axis of the graph shows the epochs, and the y-axis represents the accuracy at the corresponding epoch. Remember, the higher the reading in the accuracy graph, the better the model. The range of the accuracy is 0 to 1.
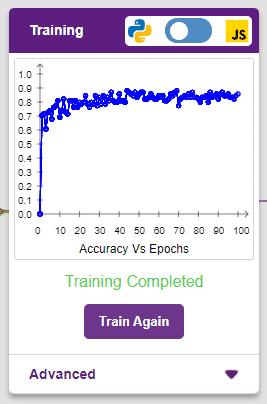
Testing the Model
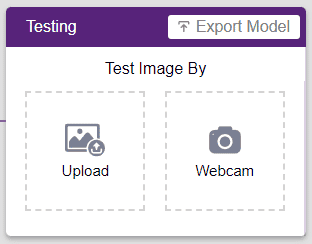
Hand Pose Classifier
The model will return the probability of the input belonging to the classes.You will have the following output coming from the model.
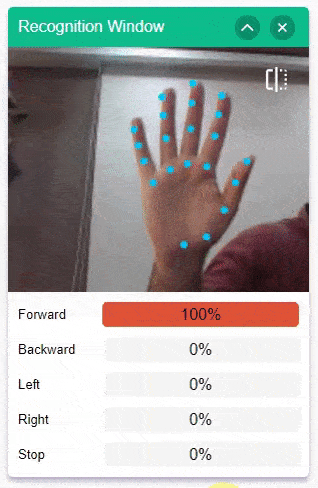
Logic
The Quadruped will move according to the following logic:
- When the forward gesture is detected – Quadruped will move forward.
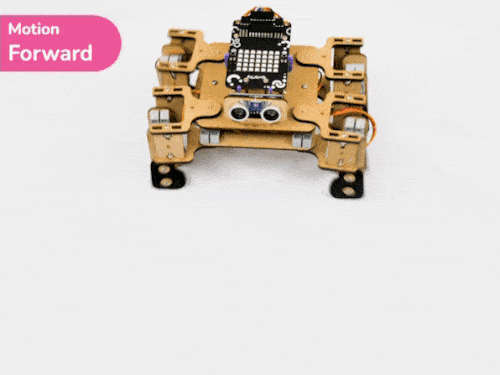
- When the backward gesture is detected – Quadruped will move backward.
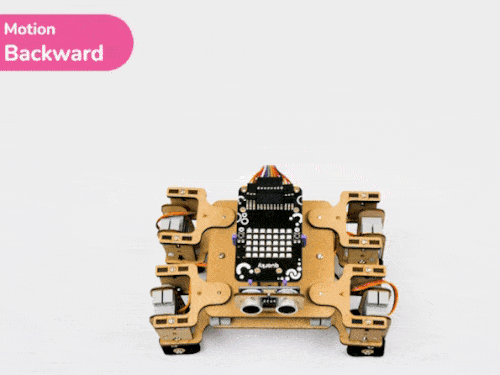
- When the left gesture is detected – Quadruped will turn left.
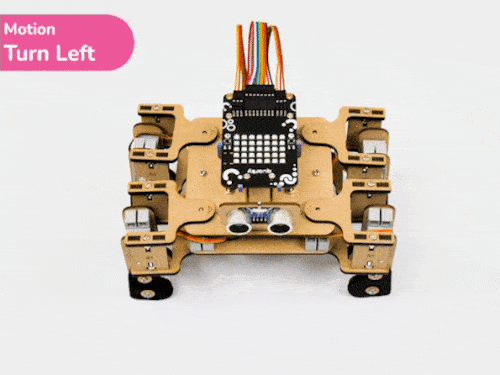
- When the right gesture is detected – Quadruped will turn right.
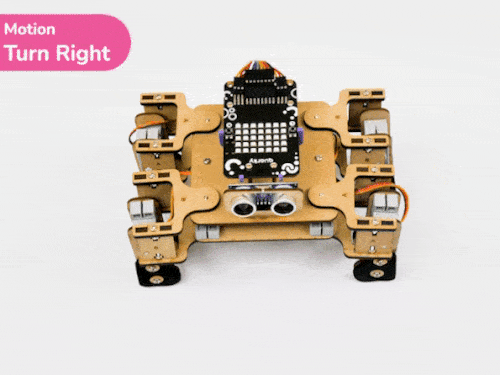
Code
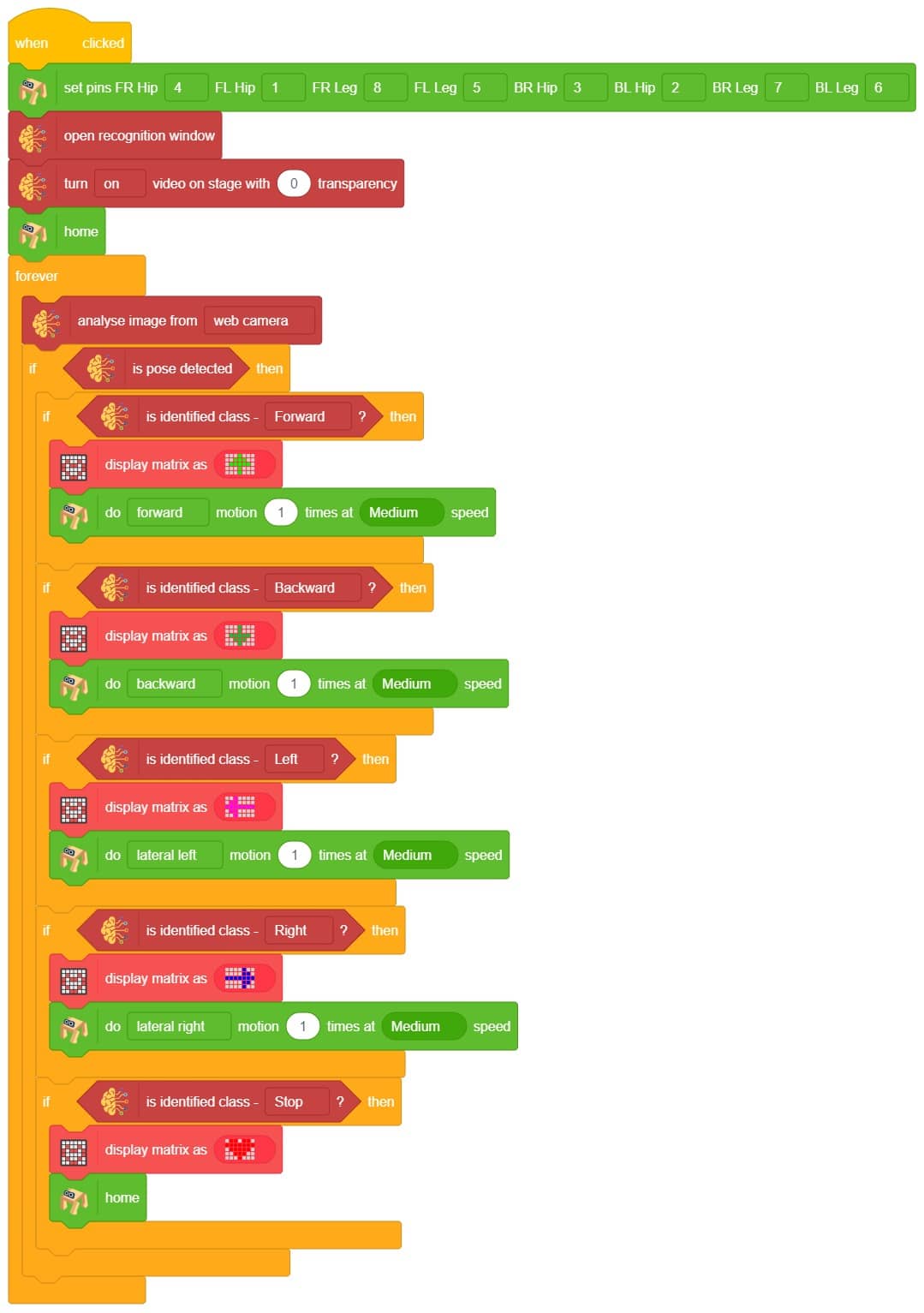
Output
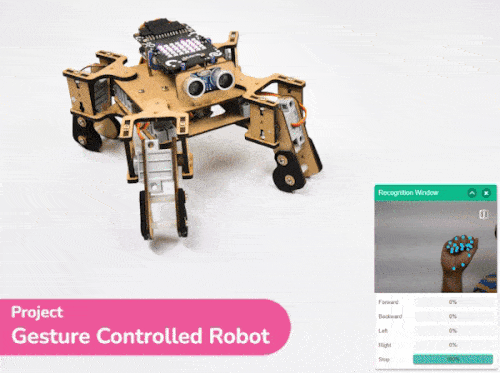